2024 Top 10 Clinical Trial Failures
Explore the top 10 clinical trial failures of 2024, key lessons learned, and implications for drug development and patient safety.
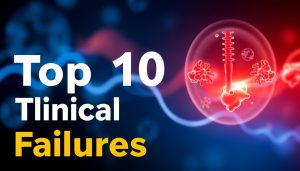
Clinical trials are the cornerstone of pharmaceutical innovation and patient safety. In 2024, numerous high-profile clinical trial failures have underscored the challenges of drug development, regulatory compliance, and patient recruitment. While these setbacks may seem discouraging, they provide invaluable lessons for the entire medical industry—from biotechnology and pharmaceutical companies to regulatory agencies like the FDA. This article examines the top 10 clinical trial failures of 2024, discusses the underlying reasons, and highlights the key takeaways that can help shape future clinical research.
Understanding Clinical Trial Failures in the Medical Industry
Clinical trial failures occur when a study does not meet its primary endpoints, encounters significant safety issues, or is halted due to poor efficacy. These failures can result from various factors, such as inadequate trial design, unexpected adverse events, or challenges in patient recruitment. In the competitive landscape of drug development, each failure offers an opportunity to refine strategies, improve research methodologies, and ensure that future trials are safer and more effective.
The Role of Regulatory Agencies and Compliance
Regulatory agencies like the FDA and EMA play a critical role in overseeing clinical research. They enforce stringent guidelines that safeguard patient safety and ensure data integrity. However, even with rigorous oversight, clinical trials can fail due to unforeseen circumstances or limitations in the current scientific understanding. Learning from these failures is essential for enhancing regulatory frameworks and fostering innovation within the industry.
Importance of Data Analysis and Patient Safety
In an era where unstructured data makes up more than 80% of healthcare information, leveraging advanced analytics and artificial intelligence (AI) is becoming increasingly important. AI-driven tools can help researchers identify patterns and predict potential failures early in the trial process, ultimately improving patient safety and the overall efficiency of drug development.
Top 10 Clinical Trial Failures in 2024
Below, we detail the ten most significant clinical trial failures of 2024, offering insights into each case and the lessons learned.
1. Case Study: CardioHeal Study
Overview:
The CardioHeal Study, aimed at testing a novel heart failure medication, was one of the most anticipated trials of the year. However, mid-trial data revealed a lack of efficacy in improving cardiac function, coupled with unexpected adverse events in a significant portion of participants.
Key Issues:
– Inadequate endpoint selection led to ambiguous efficacy results.
– Safety concerns arose due to unforeseen side effects, prompting the early termination of the trial.
Lessons Learned:
This failure highlighted the necessity for robust endpoint design and more extensive preliminary studies to gauge safety and efficacy before large-scale trials.
2. Case Study: OncoVision Trial
Overview:
The OncoVision Trial was designed to evaluate a breakthrough immunotherapy for advanced melanoma. Despite promising preclinical data, the trial failed to demonstrate a significant survival benefit compared to the standard of care.
Key Issues:
– Patient stratification was suboptimal, leading to a heterogeneous study population.
– The trial design did not adequately account for the variability in patient response.
Lessons Learned:
Refining patient selection criteria and employing adaptive trial designs can enhance the precision of immunotherapy studies and improve outcomes.
3. Case Study: NeuroGen Research
Overview:
NeuroGen Research sought to explore a new treatment for Alzheimer’s disease. The trial was halted after interim analysis indicated no improvement in cognitive function, and some patients experienced accelerated disease progression.
Key Issues:
– The biomarkers used to assess efficacy were not sufficiently validated.
– The dosage regimen was not optimized for the target population.
Lessons Learned:
This case underscores the importance of validating surrogate endpoints and tailoring dosage regimens to specific patient demographics in neurodegenerative disease research.
4. Case Study: BioThera Study
Overview:
BioThera Study evaluated a novel biologic therapy intended for the treatment of rheumatoid arthritis. The trial faced significant setbacks when an unexpectedly high rate of immunogenic reactions led to severe adverse events.
Key Issues:
– Inadequate preclinical immunogenicity assessments.
– Failure to predict the immunological profile in diverse patient populations.
Lessons Learned:
Enhanced preclinical models and more rigorous immunogenicity testing are critical for the success of biologic therapies in autoimmune diseases.
5. Case Study: RenalRestore Trial
Overview:
The RenalRestore Trial aimed at regenerating kidney tissue in patients with chronic kidney disease using stem cell therapy. Despite initial optimism, the trial was terminated due to the inability to achieve significant functional improvement.
Key Issues:
– Poor cell engraftment and viability post-transplantation.
– Insufficient control of the microenvironment necessary for tissue regeneration.
Lessons Learned:
Future regenerative medicine trials must focus on optimizing cell delivery methods and creating supportive microenvironments to enhance therapeutic efficacy.
6. Case Study: PulmoClear Study
Overview:
PulmoClear Study investigated a new inhaled drug for chronic obstructive pulmonary disease (COPD). The study did not meet its primary endpoints, with data showing no significant improvement in lung function and quality of life.
Key Issues:
– Overestimation of the drug’s bioavailability in lung tissue.
– Inadequate consideration of patient adherence to the inhalation protocol.
Lessons Learned:
Accurate pharmacokinetic modeling and robust patient adherence strategies are essential for the success of respiratory therapies.
7. Case Study: EndoBalance Trial
Overview:
EndoBalance Trial focused on a new endocrine therapy for type 2 diabetes. Although the treatment showed promise in early phases, the trial was discontinued when long-term safety data indicated an increased risk of cardiovascular events.
Key Issues:
– Delayed identification of adverse cardiovascular outcomes.
– Insufficient monitoring protocols for high-risk patients.
Lessons Learned:
Implementing comprehensive long-term safety monitoring and risk stratification is vital in trials involving chronic conditions like diabetes.
8. Case Study: GastroRelief Study
Overview:
The GastroRelief Study aimed to evaluate a novel treatment for inflammatory bowel disease (IBD). The trial was marred by inconsistent efficacy results and a high dropout rate among participants.
Key Issues:
– Variability in patient response due to genetic and environmental factors.
– Inadequate patient support and engagement throughout the trial.
Lessons Learned:
Personalized medicine approaches and improved patient retention strategies are crucial for successful clinical trials in complex diseases such as IBD.
9. Case Study: DermalCure Trial
Overview:
DermalCure Trial tested an innovative topical treatment for psoriasis. Despite positive preclinical data, the trial failed to achieve statistically significant improvements in skin lesion reduction.
Key Issues:
– Flawed endpoint assessments and measurement inconsistencies.
– Suboptimal formulation of the topical agent, reducing its efficacy.
Lessons Learned:
Standardizing assessment methods and optimizing drug formulation are key factors in dermatological clinical trials.
10. Case Study: OrthoFlex Study
Overview:
OrthoFlex Study evaluated a cutting-edge orthopedic implant designed to enhance bone healing in patients with fractures. The trial was terminated early due to high complication rates and implant failure.
Key Issues:
– Mechanical design flaws in the implant.
– Inadequate preclinical testing under stress conditions.
Lessons Learned:
Rigorous engineering validation and comprehensive preclinical testing are critical to ensuring the safety and performance of medical devices in orthopedics.
Key Lessons and Regulatory Implications
Lessons Learned from Clinical Trial Failures
Each clinical trial failure offers crucial insights for the medical industry. Key lessons include:
– Optimizing Trial Design:Proper endpoint selection, patient stratification, and adaptive trial designs are fundamental to capturing meaningful data.
– Enhanced Safety Monitoring: Implementing rigorous preclinical and clinical safety assessments can preempt adverse events and improve patient outcomes.
– Robust Data Analysis: Leveraging advanced analytics and AI can aid in early detection of issues and refine decision-making processes during trials.
– Regulatory Compliance: Adhering to stringent regulatory standards ensures data integrity and patient safety, while facilitating smoother trial processes.
Regulatory Implications for Drug Development
Regulatory bodies continually update guidelines to incorporate lessons from clinical trial failures. Enhanced oversight on trial design, patient safety, and data analysis methods is expected to drive future improvements in clinical research. For pharmaceutical companies, understanding these regulatory trends is critical for navigating the complex landscape of drug development and ensuring successful trial outcomes.
Future Directions in Clinical Research
Integrating Advanced Technologies
The future of clinical research lies in the integration of technologies such as AI, machine learning, and big data analytics. These tools can predict potential failures, optimize patient recruitment, and streamline data collection, ultimately reducing trial costs and improving efficacy.
AI-Driven Decision Support Systems
Incorporating AI-driven decision support systems into clinical trial workflows can help identify at-risk patient populations and potential safety concerns early on. This proactive approach will be invaluable in refining trial protocols and ensuring timely interventions.
Embracing Personalized Medicine
As the medical industry moves towards personalized medicine, tailoring treatment protocols to individual patient profiles becomes increasingly important. Clinical trials that incorporate genetic, environmental, and lifestyle factors are more likely to yield successful outcomes and drive innovation in drug development.
Precision in Patient Stratification
Advanced biomarkers and genetic profiling are set to play a major role in future clinical trials. By improving patient stratification, researchers can enhance the accuracy of efficacy assessments and reduce variability in trial results.
Enhancing Global Collaboration
Global collaboration among researchers, regulatory agencies, and pharmaceutical companies is essential for overcoming the challenges of clinical trial failures. Sharing data and insights across borders can lead to more robust trial designs and accelerated drug development.
Standardizing Global Clinical Practices
Efforts to standardize clinical trial practices internationally will facilitate smoother regulatory approvals and improve overall patient safety. This collaborative approach is vital for ensuring that breakthrough treatments reach patients in need more efficiently.
Conclusion
The top 10 clinical trial failures of 2024 have provided the medical industry with a wealth of lessons in trial design, patient safety, and regulatory compliance. While each failure represents a setback, the insights gained are invaluable for shaping the future of drug development and clinical research. By optimizing trial protocols, leveraging advanced analytics, and embracing personalized medicine, the industry can minimize risks and enhance patient outcomes.
Regulatory agencies and pharmaceutical companies alike must take these lessons to heart. With a focus on rigorous safety monitoring, robust data analysis, and global collaboration, future clinical trials can be designed to overcome current challenges and pave the way for groundbreaking treatments. The journey toward innovation is fraught with setbacks, but each failure brings us one step closer to delivering safer, more effective therapies that improve lives worldwide.
As we move forward, the integration of AI, personalized medicine, and enhanced regulatory standards will be key to transforming clinical research. The lessons from 2024 serve as a reminder that even in failure, there is an opportunity for growth and innovation—a chance to build a future where clinical trials not only advance medical science but also ensure the highest standards of patient care.